
The use of these approaches allows for the derivation of many more levels of detailed information, often at the pixel by pixel level, than is able to be appreciated by the human eye. As a result, a standard radiographic image such as a CT scan may be decomposed by the neural net to derive a host of characteristics which may provide valuable information.

Such data may be value in radiogenomic correlations of genetic mutations, assessment of tumor phenotype, and prediction of response to therapy or patient prognosis.
Helping to distinguish the overlapping nature of terminology, he emphasized that deep learning is a subset of machine learning, which is itself a subset of artificial intelligence. Artificial intelligence, the most broad of these approaches, encompasses any technique which enables computers/technology to mimic human behavior. In contrast, machine learning is a subset of artificial intelligence (AI) which focuses on the use of statistical technique to enable machines to improve their performance with experience. Finally, deep learning is a subset of machine learning which facilitates the computation of multi-layer neural networks.
The underlying principle of radiomics is that there is a correlation between the radiographic appearance on a medical imaging and the underlying biology. Machine learning approaches seek to utilize such imaging features to provide classification.

The development of a radiomics approach requires a multi-set pipeline:
- Relevant images must be acquired from a sufficiently large sample as to provide relevant and generalizable results
- Images must undergo segmentation
- Images must undergo registration
- Features, including signal intensity, shape, texture, and many others, must be extracted from the images
- Utilizing available clinical data, machine learning decision models must be created to generate prediction of relevant clinical data on the basis of the radiographic images.
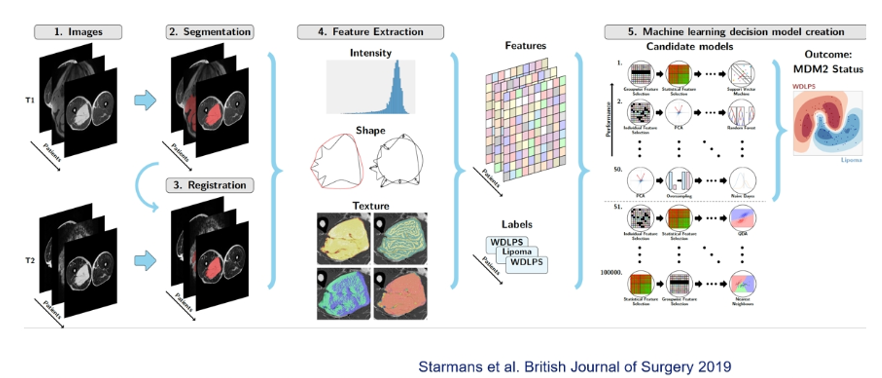
Dr. Niessen then highlighted his work which seeks to develop a classifier for patients with high-risk prostate cancer on the basis of radiomic features on multi-parametric magnetic resonance imaging (MRI). The first approach he highlighted is the use spatially matched magnetic resonance images of whole prostate specimens, allow cross-registration of the data. Doing so requires precise, and detailed, pathology to MRI image registration.

However, the prediction models that result have reasonable test properties with an area under the receiver operating characteristic curve (AUC) of 0.77 (95% confidence interval 0.66 to 0.87).
Others have taken the approach of using machine learning to predict clinically-significant prostate cancer among low-risk patients. As highlighted in a number of case examples, regions predicted to have prostate cancer according to the model often have proven cancer on biopsy.
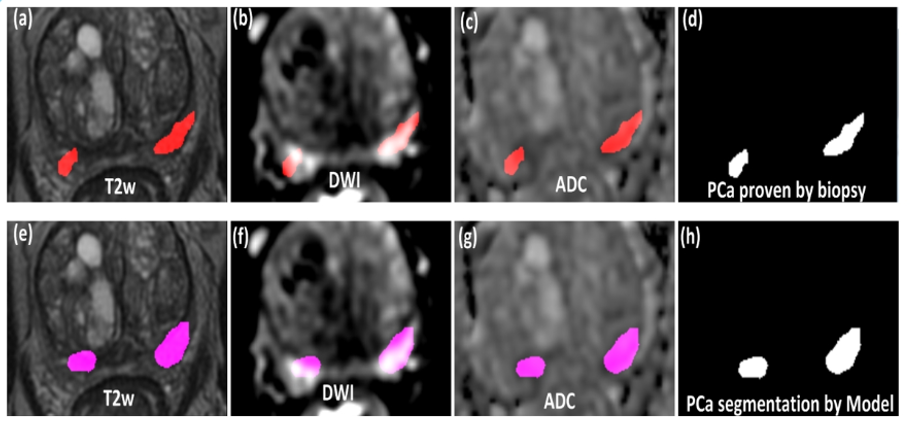
In closing, Dr. Niessen highlighted three key, take-home messages:
1. the necessity of access to high-quality multi-centre data to allow for validation and generalizability.
2. the paradigm shift of relying of imaging results for decisions that are largely driven by pathology currently
3. the potential for a “learning health care system” through use of artificial intelligence which may allow for higher quality and better accessible data and clinical decisions.
Presented by: Wiro Niessen, PhD, Erasmus, Rotterdam, the Netherlands
Written by: Christopher J.D. Wallis, Urologic Oncology Fellow, Vanderbilt University Medical Center, @WallisCJD on Twitter at the Virtual 2020 EAU Annual Meeting #EAU20, July 17-19, 2020.