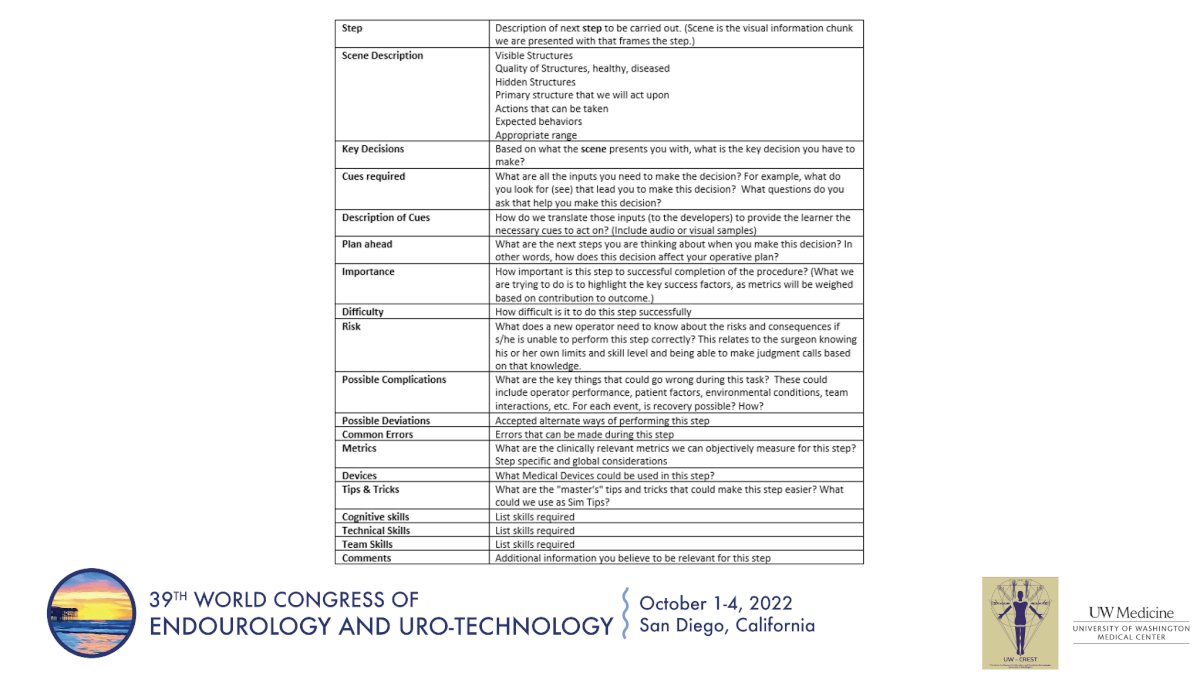
Machine Learning: Providing Us the Core Metrics
Then Dr. Andrew Hung took the stage to discuss using machine learning to identify and utilize core metrics to improve surgical simulation training. Automated performance metrics (APMs) that are derived through analyzing surgical video and robot system data, patient clinicopathological features, and surgeon factors that consist of efficiency and technical skills, were used in a survival analysis to predict patient outcomes, specifically urinary continence recovery. Dr. Hung’s group applied a deep learning model to these factors for the assessment of continence recovery and found that assessing the combination of the APMs and technical skills was superior to assessing the APMs or technical skills alone, as shown by the area under the curve in the survival analysis of 0.8, 0.6, and 0.7 respectively. Interestingly, the model also identified the most important factors leading to the prediction of continence recovery.2
Based on the promising data, Dr. Hung initiated a randomized clinical trial that examined resident performance on a lab-based vesicourethral anastomosis simulation in which all groups went through a four-week training process. One group received tailored feedback from the most relevant metrics identified by the deep learning model and compared to a group with no feedback. The results showed that those with tailored feedback improved over the control group in most parameters studied including the goal of no water leakage from the anastomosis simulation.3
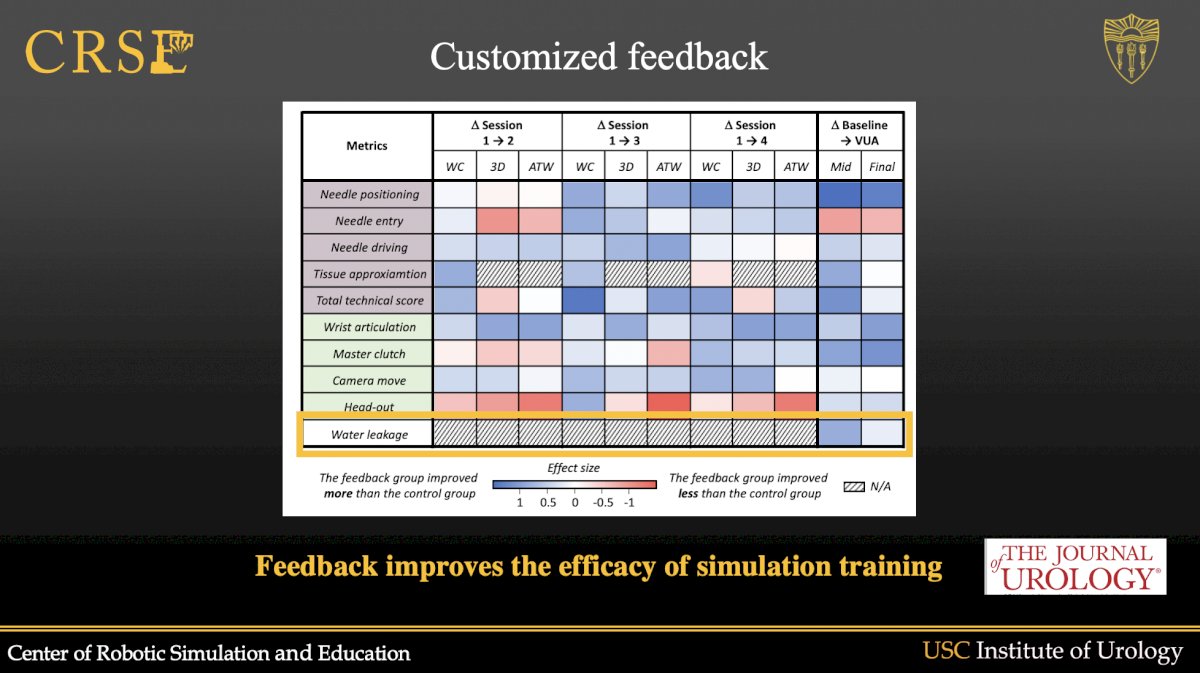
Dr. Hung then described “decoding” a robotic prostatectomy by segmenting the procedure into individual instrument maneuvers he called surgical gestures,4 with the robotic nerve sparing prostatectomy having an average of 450 distinct gestures in total. He compared the first 100 gestures of a nerve sparing prostatectomy between a group of 22 previously potent patients that did not regain erectile function at 12 months to a group of 18 previously potent patients that did regain erectile function within 12 months. The machine learning model was able to predict whether patients would regain erectile function immediately after analysis compared to waiting 12 months for that outcome feedback for the surgeon.
Needs Analysis for Patient Specific Modeling
Next, we were joined by Dr. Ahmed Ghazi who described the components and development of an effective surgical simulation model based on the experience at the University of Rochester. He described the ideal model as realistic, allowing for the complete performance of the procedure, and having potential complications occur in the setting of an error. He also stressed that there should be objective measurements of performance and care taken in the design and materials to make the model cost effective. Importantly, all the model characteristics should be agreed upon by multiple experts. Dr. Ghazi described the general workflow for the development of this type of model to include determining physician requirements, gaining expert consensus, tasking biomedical engineers to translate the requirements into a product, developing a series of prototypes that are iteratively improved, and finally product validation.
Dr. Ghazi’s then presented his group’s HoLEP/ThuLEP model developed according to the previously discussed workflow. Unique features of this model included Hydrogel that was required to replicate both laser and blunt tissue dissection including portions that are tethered by simulated adenoma or simulated blood vessels. The hydrogel was designed to be switched out for each simulation to reduce cost and an example is shown below. A global rating scale and objective measures were used to assess surgeon performance and Dr. Ghazi found significant difference between 9 experts and 49 novices in the performance metrics assessed as shown in the figure below.
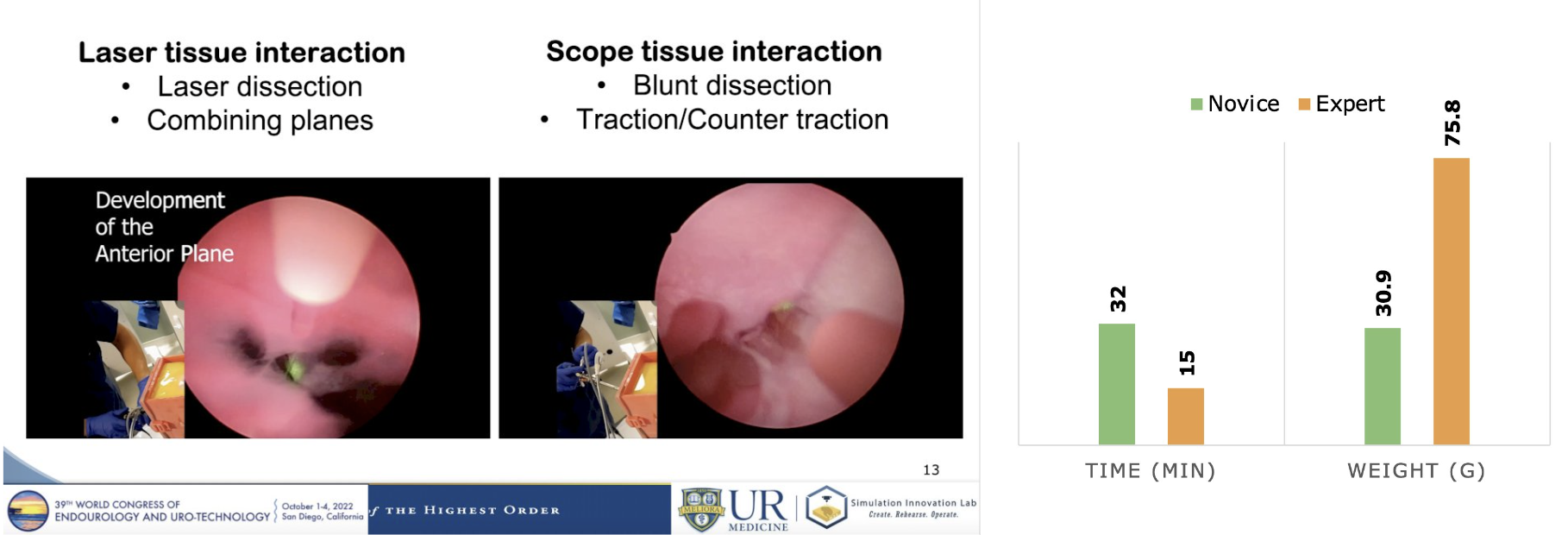
Next Dr. Ghazi discussed a PCNL model his group developed that went through a similar design process. This model had requirements of simulating the tough texture of skin and facia, the calyceal system, and access needle maneuverability. The final product consisted of a water tight distensible pelvicalyceal system containing a stone that could be lasered complete with anatomical landmarks based on prone positioning. The landmarks were palpable, US appearance was realistic, and the model did not obscure the US view of the needle. The user could feel the puncture of the needle and see efflux of urine upon successful puncture. In addition, retrograde ureteroscopy could be performed. An example of the fabrication of this model is shown below. Dr. Ghazi found differences in expert and novice performance metrics; interestingly, scores of novices increased as their use of the model increased. New on the horizon for Dr. Ghazi’s group is remote training with an expert combined with an on-site model, a supine PCNL model, and sensors implanted to measure torque.
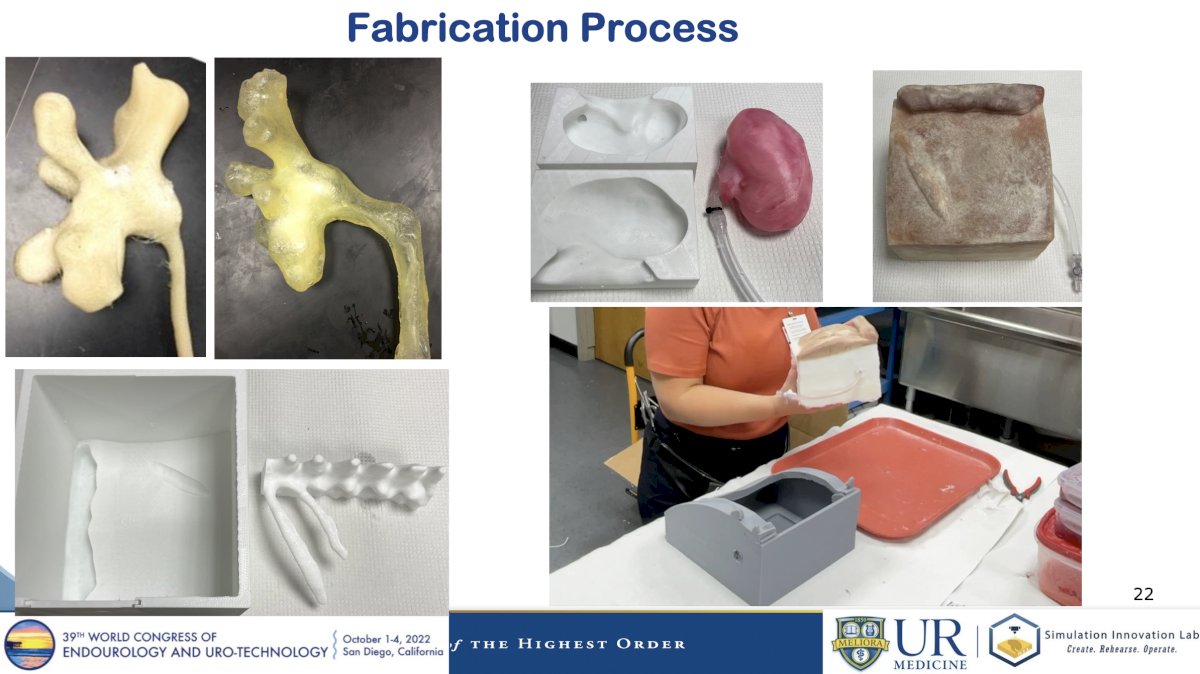
Presented by:
- Andrew Hung, MD, Assistant Professor of Urology, University of Southern California
- Lauren Poniatowski, MD, MS, Chief Resident, Department of Urology, University of Washington
- Ahmed Ghazi, MD, MHPE, FEBU, Associate Professor of Urology, Director of Simulation Innovation Lab
Written by: Zachary E Tano, MD Endourology Fellow, Department of Urology, University of California, Irvine during the 39th World Congress of Endo urology and Uro-Technology (WCET), Oct 1 - 4, 2022, San Diego, California.
References:
- Sweet, R.M., The CREST Simulation Development Process: Training the Next Generation. J Endourol, 2017. 31(S1): p. S69-s75.
- Trinh, L., et al., Survival Analysis Using Surgeon Skill Metrics and Patient Factors to Predict Urinary Continence Recovery After Robot-assisted Radical Prostatectomy. Eur Urol Focus, 2022. 8(2): p. 623-630.
- Ma, R., et al., Tailored Feedback Based on Clinically Relevant Performance Metrics Expedites the Acquisition of Robotic Suturing Skills-An Unblinded Pilot Randomized Controlled Trial. J Urol, 2022. 208(2): p. 414-424.
- Ma, R., et al., A Novel Dissection Gesture Classification to Characterize Robotic Dissection Technique for Renal Hilar Dissection. J Urol, 2021. 205(1): p. 271-275.